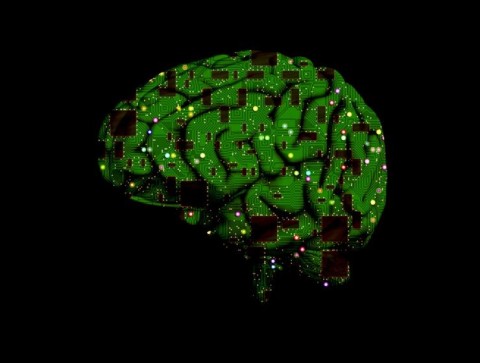
Siri knows where you live, but she couldn’t drive you there. Despite their name, artificial neural networks are very different from the brain. Yet machine learning performance could be improved if informed by state-of-the-art neuroscience.
A team of researchers from Georgia Tech and other local universities would study this problem with a grant up to $2 million, dependent on successful completion of milestones, from the Defense Advanced Research Projects Agency’s (DARPA) Lifelong Learning Machines (L2M) program managed by Dr. Hava Siegelmann. DARPA’s goal would be to develop new machine learning approaches that enable systems to learn continually while they operate and apply prior knowledge to new situations.
School of Computer Science Professor Constantine Dovrolis, Georgia Tech Research Institute Senior Research Scientist Zsolt Kira, Georgia State University neuroscience Professor Sarah Pallas, and Emory biology Associate Professor Astrid Prinz are collaborating on the two-year project.
Bringing neural networks to the 21st century
The concept of modeling a computational neural network based on the brain first arose in the 1950s, but it hasn’t evolved much since.
“Obviously, since the ‘50s there’s been a lot of progress in neuroscience, but not a lot of it has translated to machine learning,” Kira said. “Supervised machine learning through neural networks is fundamentally a computer scientist’s translation of a high-level understanding of the brain from the past. But I think there’s a lot we can learn from contemporary neuroscience.”
One of the fundamental problems of machine learning that neuroscience could alleviate is what Dovrolis calls “catastrophic forgetting.” When the artificial neural network learns a new task, it often forgets the previous one.
“Deep learning networks are very different from the brain, both in terms of structure (architecture) and function (dynamics),” Dovrolis said.
Take the brain of a baby. Within the first few years of life, it not only has the ability to learn but also to generalize with very little supervision. Dovrolis believes that machine learning can achieve the same goal but only through a major departure from the currently established machine learning paradigms.
“The brain is really the only example of general intelligence we have,” Dovrolis said. “It makes sense to take that example, identify its fundamental principles, and transfer them to the computational domain.”
Bridging the gap between neuro and computer science
It may make sense, but it’s also controversial. Many computer scientists see the brain as mere hardware, and they prefer to focus instead on more statistical machine learning approaches. This is why this project is so unique: It brings together different ideas from network science, machine learning, evolutionary computing, computational neuroscience, and systems neuroscience — fields that should’ve been working together from the start.
“It’s easier for each field to work by themselves because it’s very comfortable,” Kira said. “But there’s a lot of potential if you actually make the effort to bring people together.”
Yet working with neuroscientists doesn’t just benefit computer scientists. Many neuroscientists believe computing could help with better modeling of biological networks, and ultimately, a deeper understanding of how the brain works.
“Neuroscience can in turn be guided by results from machine learning research that can inform new experiments to deepen our understanding of the brain,” Prinz said.
One of these examples is the flexibility of the brain.
"Neural circuits in the developing brain are highly flexible and adaptable to environmental changes, which endows them with an ability to learn rapidly and to self-repair after damage,” Pallas said.
Adult brains are much less plastic, so one of the neuroscientists’ goals is to uncover the neuronal mechanism that regulates the level of plasticity versus stability in brain circuits. With this, they can harness the mechanism for medical purposes and design machines that can continue learning without forgetting.
Approaching the research
The project should attempt to address five goals of the L2M program:
• Continual learning: The building block of the cortex is a largely invariant structure referred to as a “cortical column.” The function of cortical columns is not known yet, but it seems that they act as associative memories and predictors. The structure of cortical columns suggests that recurrent neural networks could learn incrementally and in an unsupervised manner simply by interacting with the environment.
• Adaptation to new tasks/environments: These cortical columns could interconnect through deep brain-wide hierarchies and nested feedback loops that interact and inform each other, enabling the brain to adapt to different environments with minimal need for re-training.
• Goal-driven perception: At any time, the brain is receiving data from many sensory sources. Hierarchical neural networks could use task-driven inputs to adjust low-level sensory processing and integration dynamically, depending on top-down goal-related signals.
• Selective plasticity: The project should be investigating how the connections and weights between (artificial) neurons could be adjusted when a new task is encountered, without catastrophically forgetting previous tasks. Neuromodulator-driven plasticity and homeostatic plasticity are two biological mechanisms that could be transferred in machine learning to address this problem.
• Monitoring and safety: Researchers would also investigate how to ensure stability and safety, based on the organization of the brain’s autonomic nervous system. Additionally, the safety concern could be further addressed through an “artificial impulse control” system, operating on the same prediction principles as the corresponding cortical system.
This research could allow machine learning to become increasingly adaptive and continually learn, which could have vast applications. A self-driving car could be programmed in the summer, but with these principles, it could learn how to drive in previously untested winter conditions. Siri could be next.
Approved for Public Release, Distribution Unlimited
Tess Malone, Communications Officer