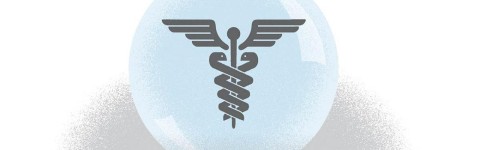
For Cassie Mitchell, predictive healthcare means using data analytics and computational approaches to best predict what care or treatment is going to work for a patient. Predictive healthcare is instrumental in identifying what Mitchell refers to as the three C’s: cause of a disease, cure simulation, and care optimization for patients.
“Predictive healthcare is just an extra, objective tool that can help the physician and patient make better decisions,” said Mitchell, assistant professor in biomedical engineering at Georgia Tech. “The analytics assist by calculating odds of diagnostic and treatment success. By ‘picking winners’ among thousands of possibilities, predictive healthcare expedites biomedical research and clinical trials, so patients get the care or cure they need, sooner.”
Mitchell’s lab is best known for taking disparate data and stitching it together “into a quilt,” she says, to identify disease patterns. Having access to millions of different measurements and data points allows her to conduct research on multifactorial diseases, or conditions caused by many contributing factors, such as Alzheimer’s Disease, Amyotrophic Lateral Sclerosis (ALS) and some cancers. Rather than isolating one factor of a disease, Mitchell’s predictive healthcare work holistically examines disease factors to predict an effective course of treatment.
Mitchell’s lab is working on a few predictive healthcare projects right now.
1
Alzheimer’s
Researchers do not know the exact cause of Alzheimer’s, though, they suspect it has to do with amyloid beta plaque buildup. Mitchell’s lab analyzed data integrated from the entire Alzheimer’s field and found that the correlation between cognition and beta plaque to be very weak. In reality, the cognitive dysfunction seen in Alzheimer’s patients is more closely related to overproduction of phosphorylated tau (p-tau), a protein that makes up the backbones of neurons. Mitchell’s data-driven research suggests that p-tau may be a better treatment target than the classic amyloid beta plaques that have been the primary focus of researchers for decades.
2
ALS
Mitchell also works on ALS, a rare neurological disease. Most ALS patients will eventually experience difficulty breathing, requiring a Bi-PAP (bi-level positive air pressure) to help the breathing muscles to do their job. The Bi-PAP is only used (and covered by insurance) when the ALS patient’s breathing capacity falls below 50 percent of normal. But Mitchell and others recognize that a patient has already succumbed to the disease at that point. There are a variety of characteristics that indicate an ALS patient is ready for Bi-PAP, and Mitchell’s lab recently showed that survival rates double if patients are put on a Bi-PAP sooner. Applying predictive healthcare to the insurance world allows patients earlier access to key interventions like Bi-PAP, and they can make better decisions about their care.
3
Leukemia
Depending on a patient’s unique disease characteristics, medical history and lifestyle, doctors can use predictive healthcare models to create a personalized medicine plan that is tailored to the patient. Cancer was one of the first fields to use personalized medicine to customize chemotherapy based on genetic-mutation profiling to maximize cancer cell killing power. However, killing cancer cells is only part of the equation. Most treatments have negative side effects, and many patients, such as those with chronic myeloid leukemia, are currently given the recommendation to stay on treatment for life.
With patients now living much longer, predicting the long-term toxicity and side effects of chemotherapy drugs is important. Mitchell is developing clinical-decision models to better predict side-effect profiles years in advance. The information can also be used to better customize a patient’s treatment options at diagnosis, during progression, or even during remission, making predictive medicine an important tool for enhancing patients’ overall quality of life.
By Georgia Parmelee